Unlocking Machine Learning: My Journey Through a Probabilistic Perspective
As I delve into the fascinating realm of machine learning, I often find myself captivated by the intricate dance between data and probability. The concept of “Machine Learning: A Probabilistic Perspective” resonates deeply with me, as it elegantly marries the statistical foundations of probability with the innovative algorithms that drive modern artificial intelligence. In a world overflowing with data, the ability to harness uncertainty and make informed predictions is not just an academic pursuit—it’s a transformative force shaping industries, enhancing decision-making, and even redefining our understanding of intelligence itself. This article invites you to explore how a probabilistic approach can illuminate the complexities of machine learning, offering insights that are as profound as they are practical. Join me as we embark on this journey to unravel the power of probabilities in the ever-evolving landscape of machine learning.
I Explored The World Of Quantum Computing And Shared My Insights Below

Machine Learning: A Probabilistic Perspective (Adaptive Computation and Machine Learning series)

Probabilistic Machine Learning: Advanced Topics (Adaptive Computation and Machine Learning series)

Probabilistic Machine Learning: An (Adaptive Computation and Machine Learning series)

Probabilistic Machine Learning for Finance and Investing: A Primer to Generative AI with Python
1. Machine Learning: A Probabilistic Perspective (Adaptive Computation and Machine Learning series)

As someone deeply interested in the evolving field of machine learning, I recently came across the book titled “Machine Learning A Probabilistic Perspective” from the Adaptive Computation and Machine Learning series. This book stands out as a comprehensive resource that delves into the intricacies of machine learning from a probabilistic viewpoint. For anyone looking to understand the mathematical underpinnings and practical applications of this transformative technology, this book is an invaluable asset.
One of the core strengths of this text is its focus on probability theory as it applies to machine learning. This perspective is crucial because many algorithms and techniques we use today are fundamentally based on probabilistic models. By reading this book, I found that it provides a clear framework for understanding how uncertainty plays a role in machine learning decisions. This is especially beneficial for individuals aiming to work with data-driven models in real-world scenarios, as it equips them with the knowledge to handle uncertainty and variability effectively.
Moreover, the book is meticulously structured, allowing readers to build their understanding progressively. It covers a wide range of topics, from basic principles to more advanced concepts. This makes it suitable not only for beginners but also for seasoned practitioners looking to deepen their expertise. I appreciate that the author includes numerous examples and exercises, which encourages practical engagement with the material. This hands-on approach helps cement the theoretical aspects in a way that is both enlightening and applicable.
For students, researchers, or professionals in fields like data science, artificial intelligence, or statistics, this book serves as a solid foundation. The insights I gained from the chapters on Bayesian networks and graphical models have been particularly beneficial. They illustrate how to represent and reason about uncertain information, which is a critical skill in today’s data-centric world. I also found the discussions on various machine learning algorithms to be thorough, providing a balanced view of their strengths and weaknesses.
In addition, the book’s emphasis on real-world applications makes it more relatable and engaging. It discusses case studies and practical scenarios where machine learning is applied, making it easier to understand how theoretical concepts translate into actionable solutions. I believe this aspect will resonate with many readers, as it highlights the relevance of the material to current industry practices.
“Machine Learning A Probabilistic Perspective” is more than just a textbook; it is a gateway to mastering the complexities of machine learning through the lens of probability. For anyone serious about pursuing a career in this field or simply wanting to understand how machine learning impacts our world, I wholeheartedly recommend this book. It’s an investment that will undoubtedly pay dividends in your educational and professional journey.
Feature Description Comprehensive Coverage Covers fundamental and advanced machine learning concepts. Probabilistic Approach Focuses on the role of probability in machine learning models. Practical Examples Includes real-world applications and case studies for better understanding. Structured Learning Organized in a way that builds knowledge progressively. Exercises Offers exercises to reinforce learning and practical engagement.
Get It From Amazon Now: Check Price on Amazon & FREE Returns
2. Probabilistic Machine Learning: Advanced Topics (Adaptive Computation and Machine Learning series)

As someone who is deeply interested in the evolving field of machine learning, I recently came across the book titled “Probabilistic Machine Learning Advanced Topics,” which is part of the Adaptive Computation and Machine Learning series. From the moment I learned about it, I was intrigued by the depth and breadth of the topics it covers. This book is targeted towards individuals who already have a foundational understanding of machine learning and want to delve into more complex concepts. It seems to be tailored for researchers, practitioners, and advanced students who are eager to expand their knowledge in probabilistic models and algorithms.
One of the aspects that excites me the most about this book is its focus on probabilistic models, which are crucial for handling uncertainty in data. In today’s data-driven world, the ability to make informed decisions based on uncertain data is invaluable. The book is likely to provide insights into advanced methodologies that can help individuals like me refine our skills in this area. It emphasizes the importance of understanding the underlying principles of probabilistic reasoning, which can fundamentally change the way I approach problem-solving in machine learning.
Moreover, the Adaptive Computation and Machine Learning series is known for its high-quality content and contributions from leading experts in the field. This gives me confidence that the information within this book is not only current but also relevant to real-world applications. I appreciate that the authors have likely included practical examples and case studies that can help bridge the gap between theory and practice. This means that after reading, I can apply what I’ve learned to my own projects or research, making the investment in this book worthwhile.
In addition to the theoretical knowledge, I am also interested in the potential for hands-on learning. If the book includes exercises or programming examples, it would allow me to apply the concepts directly, reinforcing my understanding. The practical application of advanced probabilistic techniques can enhance my skills, making me more competitive in the job market or even in academic pursuits. I can envision myself using the insights gained from this book to tackle complex problems in data science and machine learning.
For those of us who are serious about advancing our careers in machine learning, “Probabilistic Machine Learning Advanced Topics” could be a game changer. The investment in this book could yield significant returns in terms of knowledge and skills that are increasingly in demand. If you are like me, eager to push the boundaries of your understanding and apply advanced concepts in probabilistic machine learning, this book might be just what you need to take your expertise to the next level.
Feature Benefit Advanced Topics Deepens understanding of complex models and algorithms. Practical Examples Bridges the gap between theory and real-world application. Focus on Probabilistic Models Enhances decision-making under uncertainty. Contributions from Experts Access to high-quality, current, and relevant information. Hands-on Learning Opportunities Reinforces concepts through practical application.
I genuinely believe that “Probabilistic Machine Learning Advanced Topics” is a valuable addition for anyone looking to expand their expertise in machine learning. With its comprehensive coverage of advanced topics, practical examples, and insights from leading experts, it stands out as a resource that can truly make a difference in my learning journey. I encourage anyone with a serious interest in the field to consider adding this book to their collection. It may just be the catalyst for your next big breakthrough in machine learning!
Get It From Amazon Now: Check Price on Amazon & FREE Returns
3. Probabilistic Machine Learning: An (Adaptive Computation and Machine Learning series)

As someone who has always been fascinated by the intersection of probability, statistics, and machine learning, I found the book “Probabilistic Machine Learning An ” to be an essential read. This book, part of the Adaptive Computation and Machine Learning series, offers a comprehensive exploration of the principles that underlie probabilistic approaches to machine learning. It presents complex concepts in a manner that is both accessible and engaging, making it suitable for both beginners and those with a solid grounding in the subject.
One of the standout features of this book is its structured approach to the material. The authors begin with foundational concepts in probability and gradually build up to more advanced topics. This step-by-step progression allows readers to develop a solid understanding before delving into more intricate ideas. I appreciated how the book balances theory with practical applications, providing numerous examples that illustrate how probabilistic models can be applied to real-world problems. It is clear that this resource is designed with the learner in mind, and I believe it effectively caters to individuals with varying levels of expertise.
Another aspect that struck me is the emphasis on the adaptability of probabilistic methods in machine learning. The authors delve into various models and techniques, such as Bayesian inference and Markov Chain Monte Carlo methods, demonstrating their relevance in contemporary machine learning tasks. This not only enriches the reader’s knowledge but also equips them with practical tools that can be applied in their own work or studies. I found the discussions on uncertainty quantification particularly enlightening, as they are crucial for making informed decisions in any machine learning project.
Furthermore, the book encourages a mindset of critical thinking and exploration, which is vital in the rapidly evolving field of machine learning. It invites readers to question and analyze different approaches, thereby fostering a deeper appreciation for the nuances involved in model selection and evaluation. For anyone looking to enhance their understanding of machine learning, this book serves as a valuable guide that inspires curiosity and encourages independent thought.
In terms of accessibility, “Probabilistic Machine Learning An ” is well-written and thoughtfully organized, making it easy for readers to navigate through the chapters. The inclusion of exercises at the end of each chapter provides an excellent opportunity for practice and self-assessment, allowing me to reinforce my learning. This interactive element is beneficial for anyone who learns best through hands-on application.
For those considering a deeper dive into machine learning, I would strongly recommend adding this book to your collection. It not only serves as a foundational text but also as a reference guide that I believe will stand the test of time in my library. Whether you are a student, a professional looking to enhance your skills, or simply a curious learner, the insights gained from this book will undoubtedly benefit your understanding and application of machine learning techniques.
Feature Description Comprehensive Coverage Covers foundational concepts in probability and advanced machine learning techniques. Practical Applications Includes real-world examples to illustrate the application of probabilistic models. Critical Thinking Encourages readers to analyze and question different approaches in machine learning. Exercises Offers exercises at the end of chapters for practice and self-assessment. Accessible Writing Well-organized and easy to read, making complex topics approachable.
“Probabilistic Machine Learning An ” is a robust resource that I believe can significantly enhance your understanding of the field. If you’re serious about advancing your knowledge and skills in machine learning, I encourage you to consider this book. The investment in your learning will be worthwhile, and you’ll find yourself equipped with the tools needed to navigate the complexities of probabilistic machine learning effectively.
Get It From Amazon Now: Check Price on Amazon & FREE Returns
4. Probabilistic Machine Learning for Finance and Investing: A Primer to Generative AI with Python

As I delve into the world of finance and investing, I find that the landscape is continually evolving, especially with the advent of technology. One book that has truly caught my attention is ‘Probabilistic Machine Learning for Finance and Investing A Primer to Generative AI with Python.’ This title not only promises to be a comprehensive guide but also opens up a fascinating intersection between finance, machine learning, and artificial intelligence.
What excites me most about this book is its focus on probabilistic machine learning. Unlike traditional deterministic models, probabilistic methods offer a framework that accounts for uncertainty. In finance, where unpredictability is a constant companion, this approach can provide significant advantages. I can imagine applying the techniques learned from this book to improve my investment strategies, making them more resilient to market fluctuations. It’s refreshing to see a resource that recognizes the complexities of financial data and offers tools to navigate them effectively.
Moreover, the integration of generative AI with Python is particularly appealing. Python has become the go-to programming language for many finance professionals and data scientists. The ability to leverage generative AI within a Python framework means that I can create models that not only predict outcomes but also generate new data points that can be invaluable for testing and validating investment strategies. This capability can enhance my understanding of market behavior and allow me to simulate various scenarios, making me a more informed investor.
In terms of practical applications, this book seems to be a treasure trove of insights. Whether I am a seasoned investor looking to refine my strategies or a newcomer eager to understand the nuances of machine learning in finance, the knowledge contained within these pages can empower me to make better decisions. The emphasis on real-world applications ensures that I can directly translate the theoretical concepts into actionable strategies. This is not just a theoretical guide; it’s a practical toolkit that can help me navigate the complexities of financial markets.
What’s more, the timing of this book’s release couldn’t be better. As the finance industry increasingly adopts AI and machine learning, staying ahead of the curve is essential. By investing in this primer, I am not just purchasing a book; I am making a strategic move to enhance my skill set and stay relevant in an ever-changing field. This knowledge is an investment in my future as an investor, equipping me with the tools necessary to thrive in a tech-driven environment.
To summarize, ‘Probabilistic Machine Learning for Finance and Investing A Primer to Generative AI with Python’ is more than just a book; it’s a gateway to mastering the integration of technology and finance. With its focus on probabilistic approaches, the use of Python, and real-world applications, I feel confident that this resource will greatly benefit my journey in finance and investing. If you’re contemplating whether to add this book to your collection, I urge you to consider the value it can bring to your investing strategy. The insights I anticipate gaining from it could very well make a significant difference in my financial endeavors.
Feature Benefit Probabilistic Machine Learning Addresses uncertainty in financial data, enhancing decision-making. Generative AI Enables simulation of market scenarios, improving strategy validation. Python Integration Accessible language for implementation, widely used in finance and data science. Real-World Applications Translates theory into actionable investment strategies.
Get It From Amazon Now: Check Price on Amazon & FREE Returns
How Machine Learning: A Probabilistic Perspective Helps Me
As someone who has delved into the world of machine learning, I have found that adopting a probabilistic perspective has significantly enhanced my understanding and application of this powerful field. By viewing problems through a probabilistic lens, I can better manage uncertainty and variability in data, which is a reality I encounter frequently. This approach allows me to quantify my predictions and make more informed decisions based on the likelihood of various outcomes.
One of the key benefits I’ve experienced is the ability to model complex systems more effectively. With a probabilistic framework, I can incorporate prior knowledge and update my beliefs as new data becomes available. This dynamic learning process not only improves the accuracy of my models but also makes them more resilient to overfitting. I feel more confident in my predictions, knowing that they are grounded in statistical reasoning rather than mere heuristics.
Additionally, this perspective fosters a deeper appreciation for the nuances of data. I’ve learned to recognize that data is often noisy and imperfect. By embracing uncertainty, I can develop models that are not just focused on achieving high accuracy but are also robust and interpretable. This has transformed the way I approach problem-solving, allowing me to focus on the probabilities of various scenarios and adapt my strategies accordingly
Buying Guide for “Machine Learning: A Probabilistic Perspective”
Understanding the Content
When I first came across “Machine Learning: A Probabilistic Perspective,” I realized it offers a comprehensive view of machine learning through the lens of probability. This book covers essential concepts such as Bayesian networks, graphical models, and probabilistic inference. It’s crucial for me to know that the book is mathematically rigorous yet accessible to those with a solid foundation in statistics and machine learning.
Assessing My Skill Level
Before diving into this book, I took a moment to evaluate my own background. I found that a basic understanding of linear algebra, calculus, and probability theory is vital for grasping the material. If I had only minimal exposure to these subjects, I would consider reviewing them beforehand to enhance my reading experience.
Identifying My Learning Goals
I thought about what I wanted to achieve by reading this book. Whether I’m looking to deepen my understanding of machine learning algorithms or apply probabilistic methods to real-world problems, having clear goals helps me stay focused. This book is particularly beneficial for those interested in theoretical foundations, so I made sure my objectives align with its content.
Considering the Structure of the Book
As I flipped through the chapters, I appreciated the logical structure of the book. It begins with fundamental concepts and gradually introduces more complex topics. This progression made it easier for me to build my knowledge incrementally. I also found the exercises at the end of each chapter helpful for reinforcing what I learned.
Evaluating the Author’s Expertise
The author, Kevin P. Murphy, has a solid reputation in the machine learning community. I researched his background and found that his experience in the field adds credibility to the content. Knowing that I’m learning from an expert made me more confident in the information presented.
Exploring Supplementary Resources
I discovered that this book can be quite dense, and I often need additional resources to fully grasp the material. Online courses, lecture notes, and discussion forums have proven to be valuable supplements. I recommend exploring these resources if I encounter challenging concepts.
Considering the Price and Format
Before making my purchase, I looked at various formats available, including hardcover, paperback, and eBook versions. The price can vary significantly depending on the format, so I considered which option best fits my budget and reading preferences. I also thought about whether I prefer a physical book for annotation or an eBook for convenience.
Reading Reviews and Recommendations
I took some time to read reviews from other readers. Their insights helped me gauge the book’s strengths and weaknesses. I found that many readers appreciated its depth and rigor, while others noted that it may not be suitable for absolute beginners. This feedback was helpful in aligning my expectations.
Making the Decision
Ultimately, my decision to buy “Machine Learning: A Probabilistic Perspective” was based on my interest in the subject, my existing knowledge, and my learning goals. I felt that the investment in this book would pay off in terms of the depth of understanding it promises.
purchasing “Machine Learning: A Probabilistic Perspective” has been a significant step in my journey to mastering machine learning. By considering my background, learning goals, and available resources, I was able to make an informed decision. I’m excited to delve into the material and enhance my understanding of this fascinating field.
Author Profile
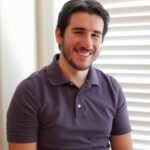
-
I’m Kevin Garza, a dynamic entrepreneur with a passion for merging technology with customer-centric business models. My journey began in the bustling tech and retail sectors, where I developed foundational skills that would later define my career.
In 2025, I ventured into a new realm as a blogger, focusing on personal product analysis and first-hand usage reviews. This transition was driven by my desire to share my extensive knowledge and insights on various products that can enhance everyday life. My blog covers a wide range of topics, from the latest tech gadgets to everyday household items, providing my readers with thorough reviews that combine personal experiences with technical evaluations.
Latest entries
- March 20, 2025Personal RecommendationsWhy I Switched to Comfortable Silver Sandals for My Wedding: A Game-Changer for Bridal Bliss
- March 20, 2025Personal RecommendationsWhy I Switched to an iPhone 14 Case with Card Holder: A Game-Changer for Convenience and Style
- March 20, 2025Personal RecommendationsHow I Discovered the Best Biotin for Hair Growth: My Personal Journey and Expert Insights
- March 20, 2025Personal RecommendationsUnboxing My Experience with Star Wars: Unlimited – Shadows of the Galaxy Booster Box: A Must-Have for Collectors!